Analog Quantum Convolutions for Advanced Image Classification
using D-Wave Advantage2 to reach quantum advantage
04.04.2025
As quantum processors continue to expand their computational capabilities, they open new possibilities for tackling real-world problems involving complex, high-dimensional datasets such as those found in financial forecasting and healthcare diagnostics. Leveraging these systems to encode and process such data is especially promising when we make use of computationally hard regimes that are beyond the reach of classical methods.
At Kipu Quantum, we are actively exploring how to apply advancements in commercially available quantum hardware, particularly the fast-annealing regime of D-Wave Advantage2, to industry-level use cases. By encoding image datasets into this architecture and generating quantum-convolved representations through quantum convolutions, we aim to boost the performance of classical machine learning models. In doing so, we extend demonstrations of quantum supremacy in quantum simulations to quantum-advantage level applications in machine learning.
Analog Quantum Convolutional Neural Networks (AQCNN) leveraging quantum quench dynamics
In contrast to our previous work on Digital-Analog Quantum Convolutional Neural Networks (DAQCNN), which was based on neutral atom hardware, we now develop a new approach to operate on analog superconducting quantum architectures. Specifically, we adapt our algorithm to leverage the fast-annealing regime in the D-Wave Advantage2 quantum processor using quantum quench dynamics. With access to over 1,200+ qubits on this system, we can encode and process large-scale datasets directly on the quantum hardware.
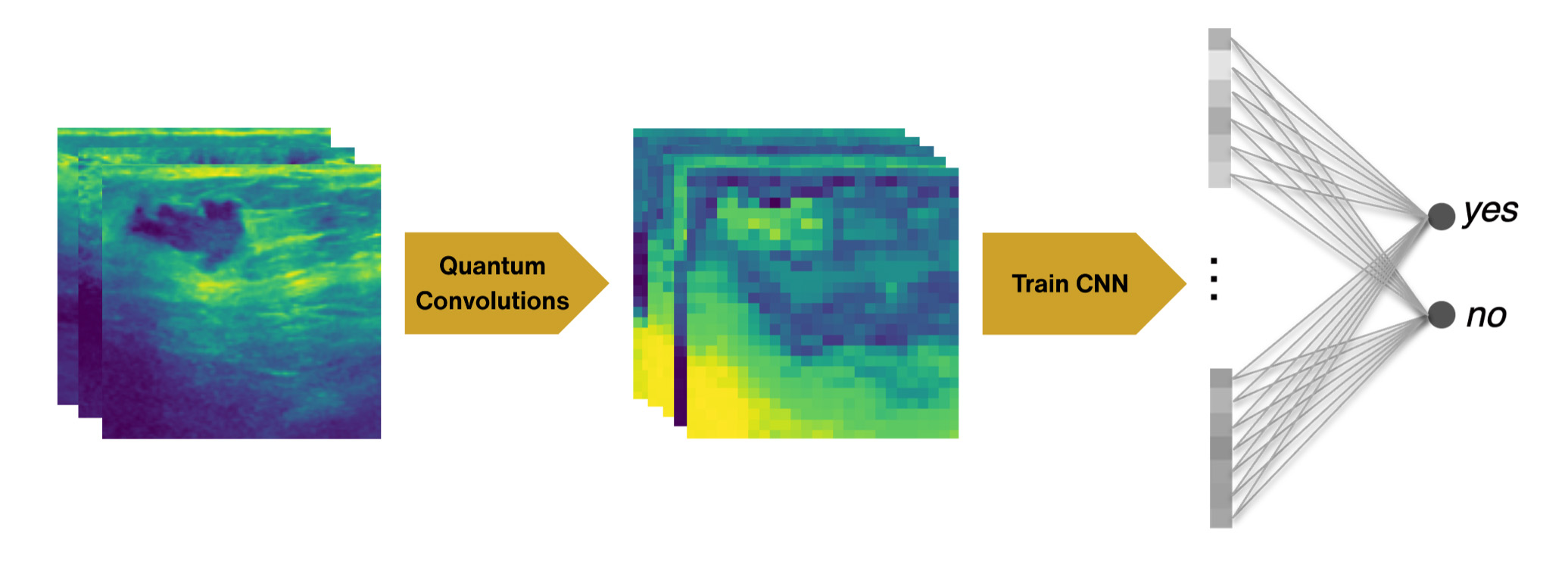
Our current implementation applies quantum convolutions to classical image datasets by first encoding pixel information, like intensities, into spin-glass Hamiltonian and then evolving the system under quantum quench dynamics—a regime where quantum entanglement, a phenomenon difficult to simulate classically, is maximized. The result is a set of quantum-convolved image representations that, when used to train classical Convolutional Neural Networks (CNNs), leads to significantly improved model performance.
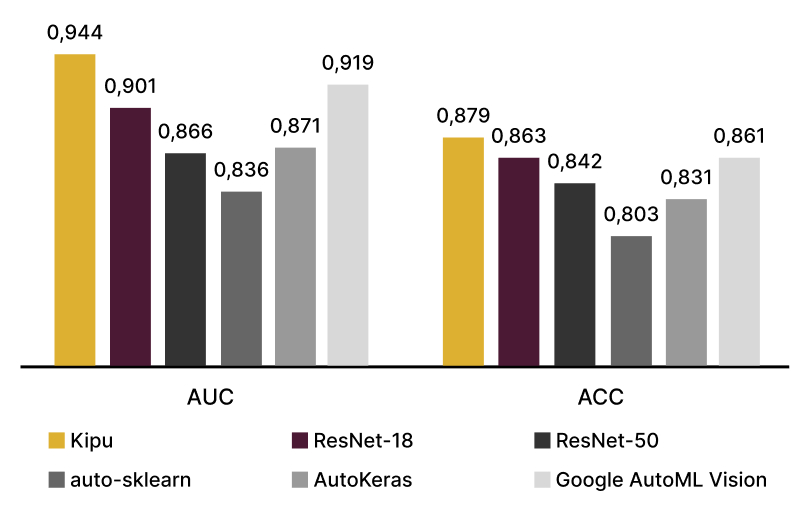
On benchmark datasets for breast cancer classification, our approach has demonstrated significant improvements over state-of-the-art techniques. Compared to leading classical algorithms, our method reduced training parameters by a factor of 1,000, improved the Area Under Curve (AUC) by 2.72%, and increased accuracy (ACC) by 1.85% when using analog quantum convolutions on D-Wave Advantage2. The reduction in the number of parameters is achieved by replacing parameterized convolutions with non-parameterized quantum convolutions. To this end, we implement parallel convolutions, each using 10s of qubits, on a 1200+ qubit chip. For higher-resolution images, we estimate to use convolutions requiring 100s of qubits, bringing us to the level of quantum advantage in this use case.
To ensure the robustness of our results, we applied statistical evaluation techniques, including proportional representation of each class across multiple folds and repetitions. This careful validation helps guarantee an unbiased and reliable measure of the generalization capabilities of our model.
Broad Applicability
The application of our AQCNN technique is not limited to image datasets. Its flexibility allows it to be effectively applied across different industries. For example, our methods could capture correlations in time series datasets like stock market data, enhancing predictions by analyzing complex interactions among different variables and temporal points.
One major benefit of AQCNN is its seamless integration with existing classical machine learning models. This compatibility ensures practical usability and immediate applicability, making superior quantum solutions available today across various domains, such as finance, science, and industrial settings. We encourage researchers and industry practitioners interested in exploring this innovative approach to collaborate with us, validate our findings, and further enhance these quantum-based methods.
Looking ahead, Kipu Quantum is committed to continually demonstrating practical quantum advantages across various industries. Over the coming weeks and months, we anticipate sharing more results that highlight the effectiveness and broad applicability of our quantum computing solutions.