Feature mapping and industrial machine learning applications
at quantum-advantage level on D-Wave’s fast annealing regimes
25.03.2025
As advances of quantum processors start to display quantum-supremacy capacities, there is huge potential in the smart encoding of computational tasks where complex quantum dynamics can offer practical advantages over classical methods. In particular, real-world applications involving high-dimensional, complex data—such as those encountered in drug discovery and medical diagnostics—present promising opportunities for exploring the capabilities of near-term quantum systems. Among the various paradigms we are considering, the use of complex quantum dynamics of commercial quantum hardware for data representation is a compelling direction, especially when grounded in computationally hard regimes that are difficult to simulate classically.
Inspired by this idea, at Kipu Quantum, we are actively exploring the practical implications of the recent demonstration of computational supremacy in quantum critical dynamic susing D-Wave Advantage 2, with a focus on real-world machine learning applications. By encoding datasets into those quantum processors—using 200+ qubits for molecular toxicity classification, a key task in early-stage drug design, and 111+ qubits for predicting myocardial infarction complications—we show that quantum-generated feature representations can significantly boost classical machine learning performances. This effectively transforms a computational quantum supremacy claim into a practical quantum advantage, providing quantum computing solutions for today’s industry needs.
Feature mapping utilizing quantum quench dynamics
In our recent work, we have developed an approach that encodes statistical patterns from classical datasets into complex spin-glass Hamiltonians, subsequently evolving these quantum systems coherently through quantum quench dynamics. This evolution drives the system into highly entangled quantum states, creating rich and complex feature representations capable of capturing intricate data correlations that are challenging to replicate classically.
Our results show that this analog quantum feature mapping through quantum quench dynamics significantly boosts the performance of classical machine learning models on two challenging benchmark datasets. We compared our method against state-of-the-art classical approaches using multiple evaluation metrics, demonstrating superior performance at the quantum-advantage level. In addition to directly employing quantum-generated features with classifiers like Gradient Boosting, we further demonstrate the applicability of these quantum feature maps within kernel-based methods, such as Support Vector Classification (SVC).
To evaluate statistical robustness, we employ Stratified Cross-validation across two distinct models, randomly shuffling the dataset and splitting it multiple times into stratified folds. This process ensures proportional representation of each class in every fold and across repetitions, resulting in a more reliable and unbiased estimation of the models' generalization performance.
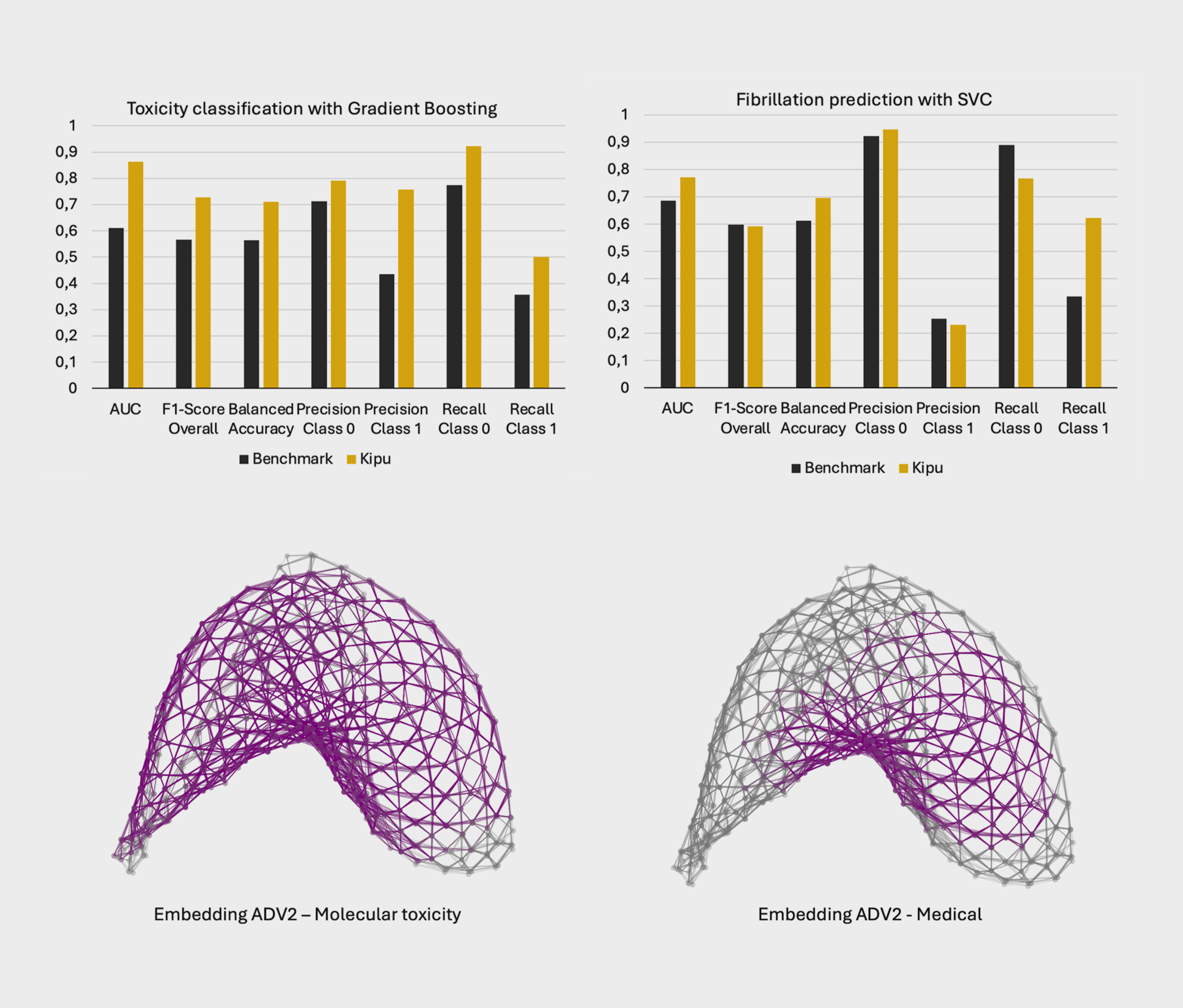
For the medical dataset, leveraging the quantum feature maps, the model achieves an AUC improvement of 12.6%, a 13.6% enhancement in balanced accuracy, and a remarkable 85.9% increase in recall for the positive class —a critical metric for improving true positive rates in medical applications compared to the purely classical model. In the molecular toxicity dataset, the model with quantum feature maps shows a 41.0% boost in AUC, a 28.2% increase in F1-Score, and a 74.0% improvement in precision for the positive class. The recall for the positive class improves by 40.0%. These consistent gains demonstrate that quantum feature maps from the quench dynamics are more effective in capturing complex patterns.
Broad Applicability
This quantum feature mapping approach is not limited to the presented use-cases—it can be applied across a wide range of domains in science, technology, and industrial applications. One of the most promising aspects of this novel Kipu Quantum technology is its compatibility with many existing classical models: when classical performance improves, applying quantum feature mapping on top consistently leads to further gains. Utilizing current quantum processors with demonstrated quantum supremacy capacities to tackle real-world problems is a crucial step toward achieving practical quantum advantage now, as they unlock the full potential of current quantum computers in meaningful applications. We are excited about the potential of these tools and warmly invite researchers, practitioners, and enthusiasts to connect with us if they are interested in exploring our datasets or models to further validate, enhance, or build upon our findings.
We are continuously proving that Kipu Quantum technologies are systematically delivering quantum-advantage level solutions. We will go ahead displaying impressive results next weeks and months, where our quantum computing products and solutions showcase quantum-advantage level for a variety of industries.