Figure 1. Example of a random sequence (top) compared to the optimal sequence of length 30 (bottom). The optimal sequence, with low autocorrelation, has a dominant peak with respect to the sidelobes, unlike the random sequence.
Quantum Optimization Benchmark Library: "The Intractable Decathlon"
16.04.2025
Combinatorial optimization problems play a crucial role across diverse industries such as logistics, finance, pharmaceuticals, telecommunications, and many others. They enable effective decision-making and resource allocation. But as these problems grow in complexity and size, classical computational methods often struggle, becoming impractical or inefficient. Quantum computing emerges as a promising solution, with the potential to handle such problems beyond classical capabilities.
Recently, as part of the Quantum Optimization Working Group, a community that includes optimization experts from companies such as Kipu Quantum, IBM, NTT, E.ON, among others, and several academic institutions, we took part in a major benchmarking initiative detailed in our latest article, "Quantum Optimization Benchmark Library: The Intractable Decathlon" [1]. This initiative aims to systematically benchmark quantum and classical optimization algorithms fairly and rigorously, identifying promising problem classes and tracking progress towards achieving quantum advantage.
Among the ten challenging optimization problems featured, the Low-Autocorrelation Binary Sequence (LABS) problem stands out for its exceptional complexity. Even for problems involving just 67 binary variables, an optimal solution remains unknown, posing a significant challenge for classical solvers.
Why LABS Matters: A Real-World Impact
Minimizing autocorrelation is critical in applications like radar systems, digital communications, and coding theory. For example, radar systems transmit binary signals and analyze their reflections. High autocorrelation leads to interference and false detections, whereas low autocorrelation enhances signal clarity and accuracy. LABS optimization specifically seeks sequences that minimize this off-peak autocorrelation, improving performance in numerous practical scenarios (see Fig. 1)
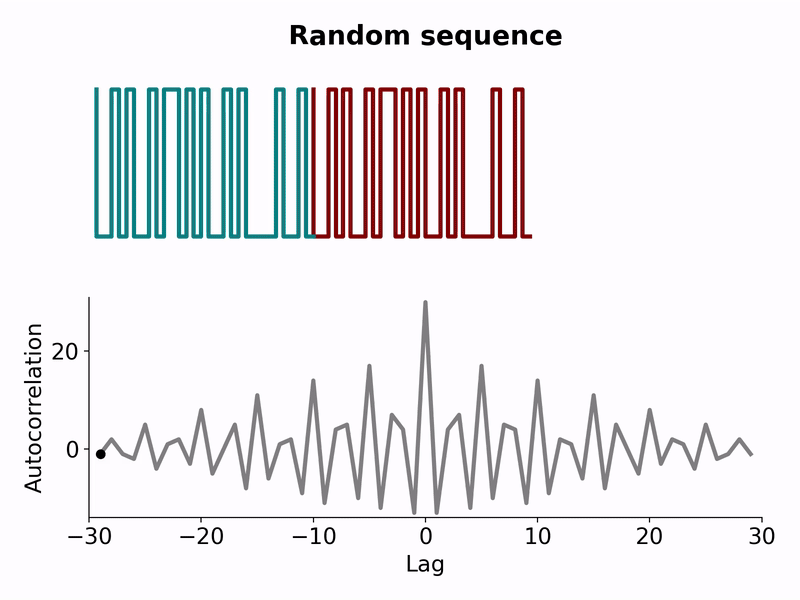
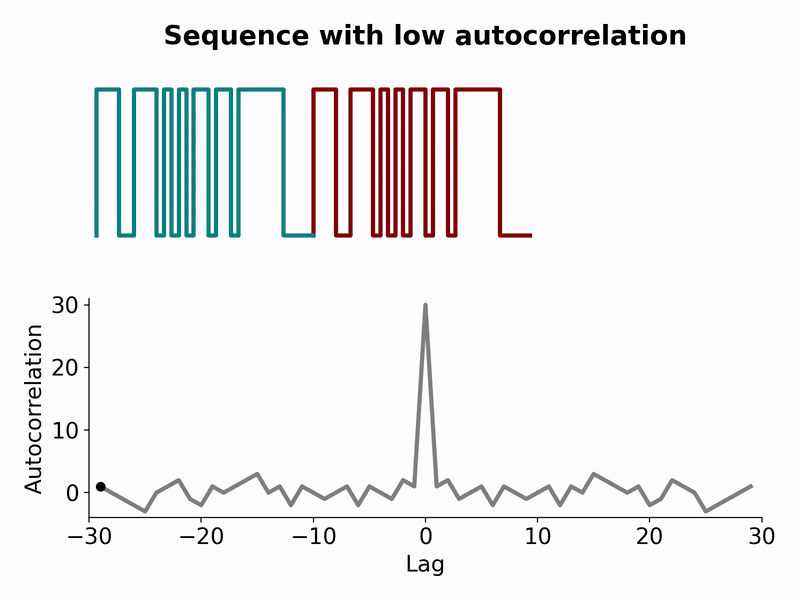
Kipu Quantum’s Contribution: BF-DCQO and Potential for Quantum Advantage
At Kipu Quantum, we have actively engaged with the LABS problem using our Bias-field Digitized Counterdiabatic Quantum Optimization (BF-DCQO) algorithm [2]. Our results demonstrate significant scaling advantages over established commercial exact solvers like CPLEX (1.73N) and Gurobi (1.61N), achieving a promising scaling factor of approximately 1.26N for sequence lengths up to N = 30. Moreover, we compared our method to the Quantum Approximate Optimization Algorithm (QAOA) with 12 layers. QAOA has demonstrated a scaling advantage over the best classical algorithm for LABS, namely Memetic Tabu Search [3]. Impressively, BF-DCQO achieved performance comparable to that of the twelve-layer QAOA while requiring 6x fewer entangling gates. This remarkable efficiency and resource reduction highlight the practicality of our approach for current and near-term quantum hardware.
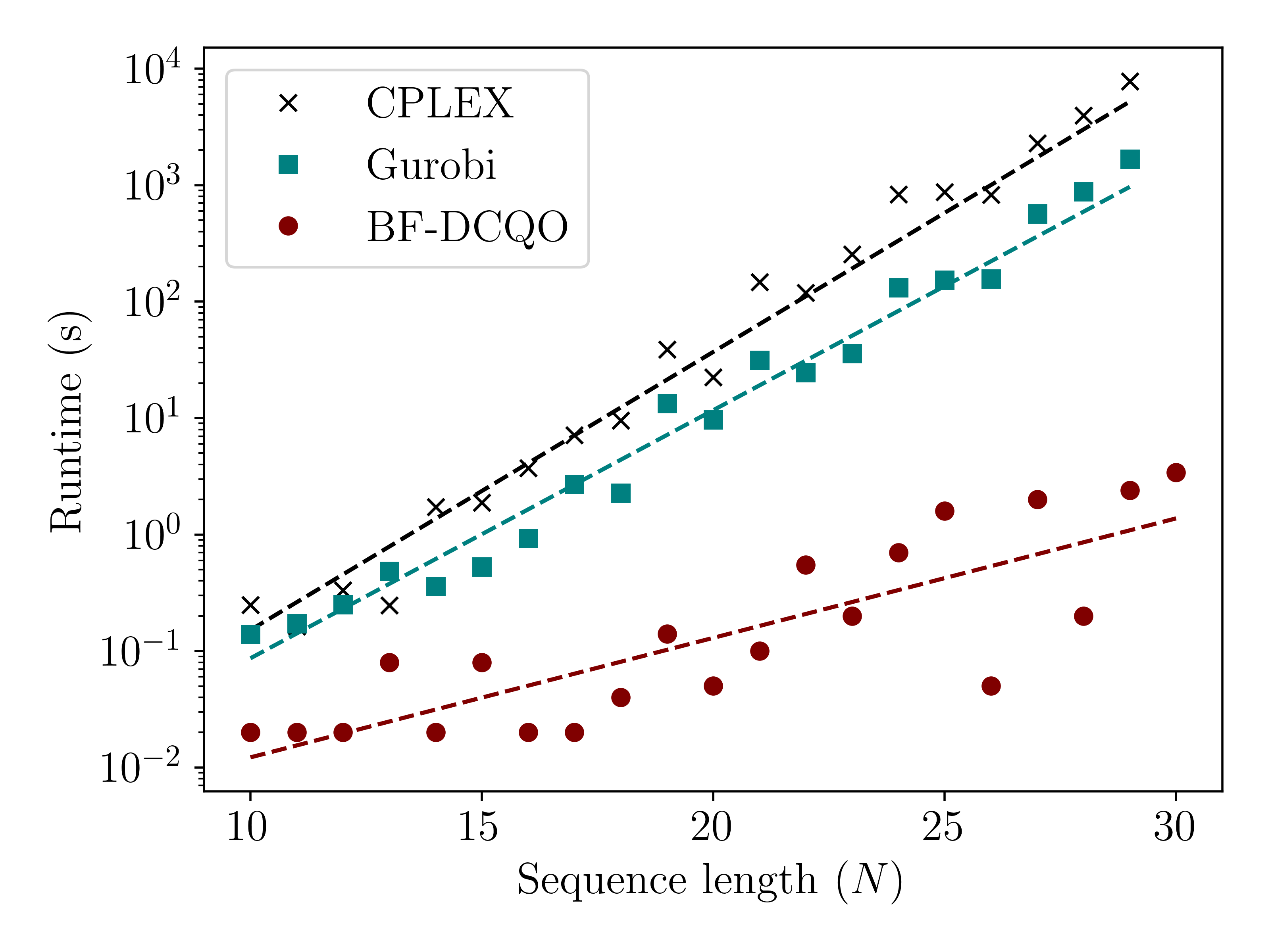
Figure 2. Runtime scaling of CPLEX and Gurobi compared to BF-DCQO for sequence lengths up to 30.
Setting New Quantum Benchmarks on Quantum Hardware
Our experiments pushed the practical quantum computing frontier forward, successfully solving LABS instances up to 20 qubits on IBM’s quantum hardware. This achievement sets a new benchmark, surpassing the previous record of 18 qubits held by the JPMorgan team using Quantinuum’s system [3]. One remarkable aspect of BF-DCQO is that it bypasses the need for variational classical optimization entirely, significantly simplifying its implementation and making it highly suitable for early-stage quantum hardware.
Looking Ahead
This collaborative benchmarking effort underscores the potential of quantum computing in addressing challenging optimization problems and accelerates the journey toward quantum advantage. At Kipu Quantum, we continue refining our algorithms, extending the scope of our research, and encouraging the broader community to explore and contribute to this exciting and rapidly evolving field.
References
[1] Koch, Thorsten, et al. "Quantum Optimization Benchmark Library--The Intractable Decathlon." arXiv preprint arXiv:2504.03832 (2025).
[2] Romero, Sebastián V., et al. "Bias-field digitized counterdiabatic quantum algorithm for higher-order binary optimization." arXiv preprint arXiv:2409.04477 (2024).
[3] Shaydulin, Ruslan, et al. "Evidence of scaling advantage for the quantum approximate optimization algorithm on a classically intractable problem." Science Advances 10.22 (2024): eadm6761.